We held our first virtual hackathon!
Form teams, show off your skills on REAL projects and win prizes.
AIHack is Imperial College Data Science Society’s flagship event. It aims to bring the most forward-thinking and creative student data scientists to solve some of the world’s most pressing challenges. Despite the pandemic, our 2021 edition was held successfully, attracting around 150 participants in 50 teams. These people were students from 20 universities, 6 countries and studying subjects ranging from Engineering, Computing to Medicine. Everyone who attended were passionate about data science and using their technical skills to solve problems in the real world. We attracted talent in different stages of their student career but we were happy to have attracted over 40% of the attendance comprised of masters and PhD students. Our Terabyte sponsor Shopee, an ecommerce platform, had the opportunity to advertise their internship programme to our members via our general channels and also speak to the hackathon participants virtually.
From a planning standpoint, even though we did not have to book a venue and handle catering or any other in-person complications, we were new to the logistics of holding a virtual hackathon. We had to use a multitude of platforms such as Discord, Devpost and Microsoft Teams to handle all the logistics of the event, which admittedly was tough.
After distributing the datasets, the participants quickly started working on the analysis, towards the goal of having a good model and solving the problem posed. Throughout the hackathon, we also organised small data science Kahoot quizzes (with prizes) and Among Us games for our participants to get their mind away from the project for a short while.
We would like to congratulate all our winners on completing these challenging tasks in a span of one and a half days! All of them had to produce some analysis, record a video and document the steps which led to their conclusions. As a result, they gained
- Chance to level up your CV for the career goals you aspire towards!
- A chance to win part of our £2000 worth of prizes.
- The experience of obtaining professional mentorship during our event.
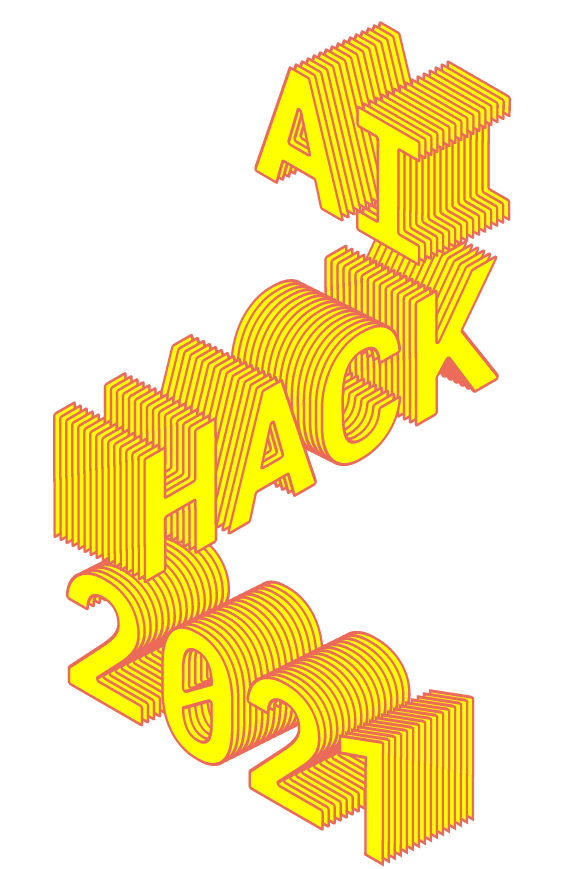
The Challenges
Boston Housing Challenge
First studied by Harrison and Rubinfeld (1978), the Boston Housing dataset has been extensively used in testing new machine learning models against existing benchmarks. It is a small dataset with only 506 observations, but is inherently interesting because a lot of things could be studied about this dataset. For this challenge, participants are free to pose their own studies and encouraged to use alternative datasets.
Data: The original Boston housing dataset can be accessed via the sklear API
A corrected version with town names and spatial information is also available here, which is augmented with longitude and latitude of the observations and corrected for the censoring error. In particular, the censoring error refers to the fact that in the original dataset, the house price is capped at USD 50,000, with values higher than this number set to USD 50,000.
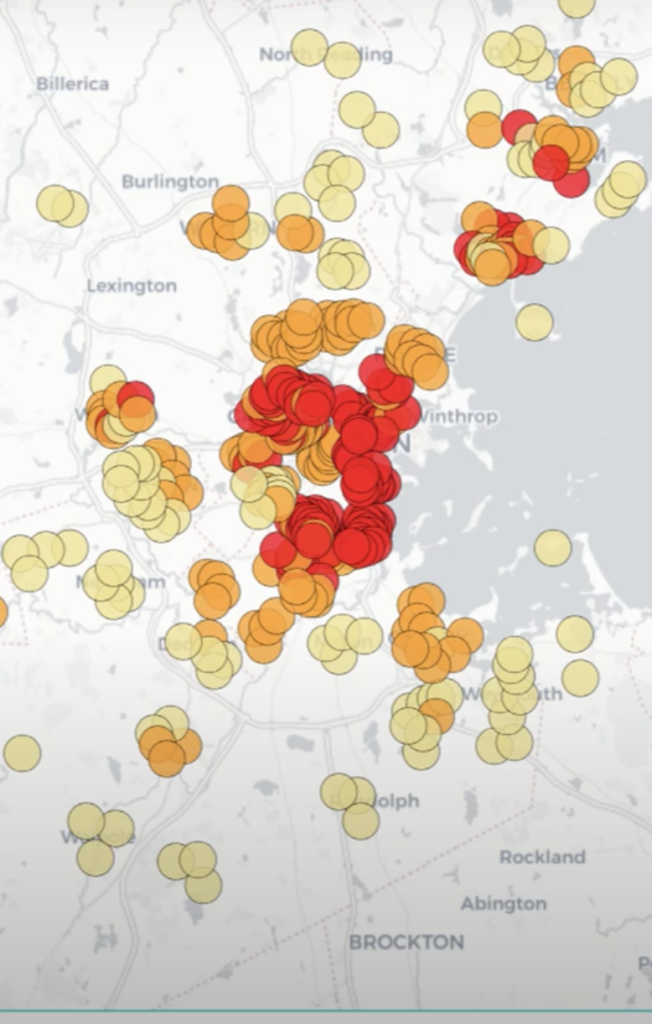
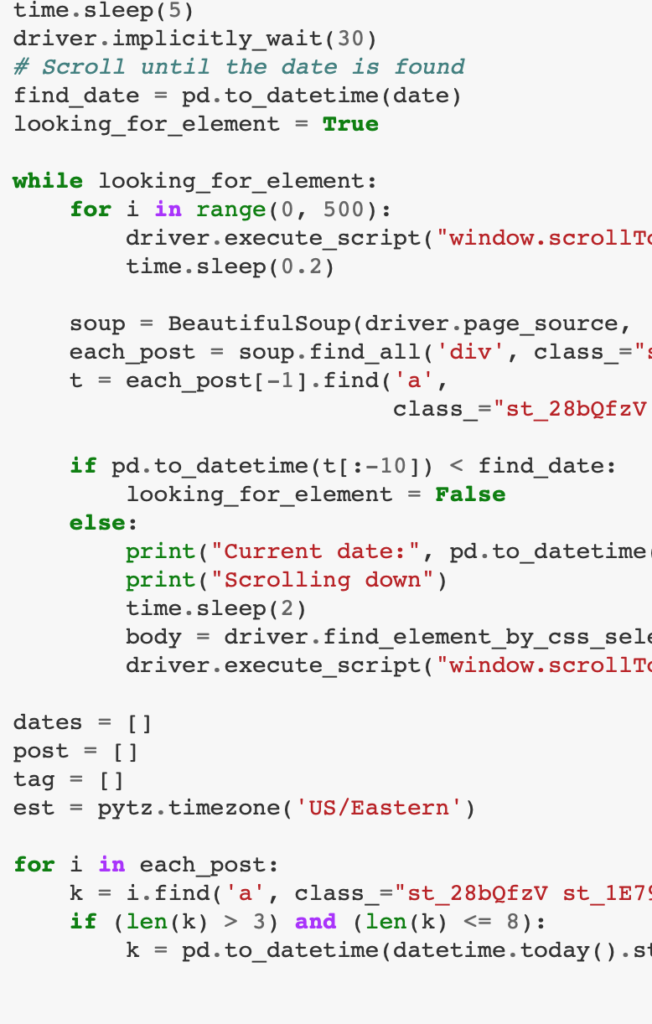
Kaiko Cryptocurrency Challenge
One or two months of minute cryptocurrency trading data for spot trading e.g. Bitcoin to dollars and derivative trading e.g. Futures. You should read up on these briefly before starting! Tackling this dataset would give you hands on experience in dealing with problems in Financial Technology. The ultimate goal is to come up with a benchmarked trading strategy. But if you don’t get that far, we are looking for useful insights/ stylised facts regarding performance (see next page) and perhaps a predictive model.
Some ideas:
- Using orderbook imbalances at various depths to predict price returns
- Analyzing statistical anomalies (e.g. abnormal returns on weekends, nights, etc.)
- Using Google Trends with specific key words to predict prices
- Explore arbitrage opportunities
2nd: Student Ensemble
3rd: Hack’em – BITweets
Crop Yield Challenge
For this challenge, participants will be tackling one of the world’s most important challenges: modelling crop yields. Climate change is having a big impact in global food security, whilst Earth’s population, in particular, in the developing world, continues to grow. Extreme weather events can have significant impacts on crops and there is (significant evidence) showing that, recently, extreme events have become (1) more extreme and (2) more frequent, making crop yield modelling a useful tool for policy makers and suppliers who are hoping to mitigate these devastating risks.
From a machine learning and statistical perspective, crop yield modelling is a challenging task that can be seen as a weakly supervised learning or multiple instance learning problem. For every year and census region (e.g. county), we can gather an abundance of features such as daily temperature, vegetation indices and soil moisture, but we only have access to 1 crop yield label.
2nd: Import torch as tf
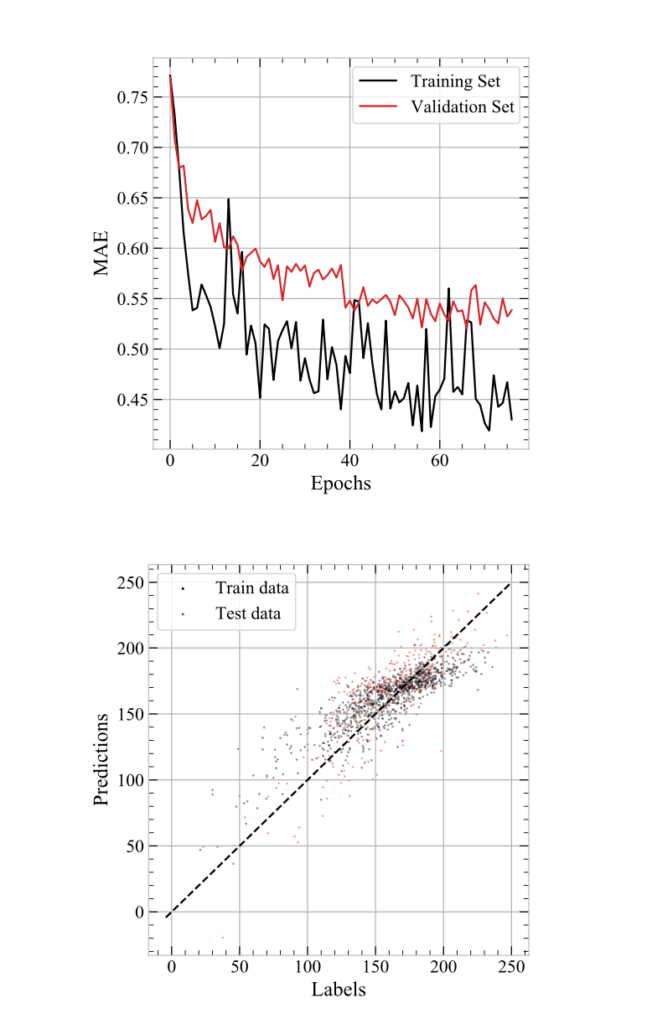
Watch Our Opening Address
We look forward to seeing everyone in the next year!